By Breno HASPARYK ANDRADE, Ferdy LARROTTA RUIZ, Tran Phuong Uyen NGUYEN, Nour ABBOUD
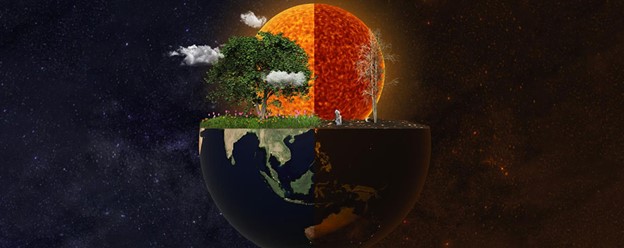
As we travel through the Earth, we can notice the symphony we hear all around, from the smallest grain of sand, to the faraway planets, to a flower putting roots in the ground, every bird in the sky, every rock, and every raindrop as it falls from the clouds, every ant, every plant, every breeze, and all the seas contribute to this beautiful composition. However, within this natural orchestra, there’s a dangerous problem that demands our attention — ‘Global Warming’.
With the variation of the temperature over recent decades, the agriculture is considered as one of the most affected sectors by global warming. These environmental changes have disrupted the agricultural landscape, affecting both its ecology and economy.
Describing the impact of global warming on agriculture is a bit like exploring a big, diverse world map. Each country in this map has its own distinct climate, crops, and economic structures. This diversity is what makes our world interesting but also makes problem-solving a bit challenging.
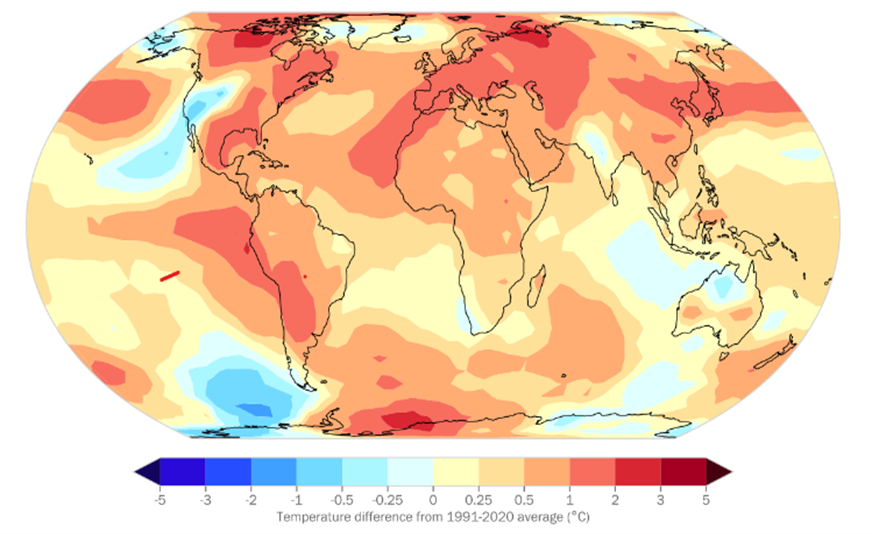
This is where the idea of assessing vulnerability for countries takes center stage. Consider Vulnerability as a measure of how susceptible a country is to the challenges posed by global warming in the sector of agriculture.
Some countries may be more resilient, while others may face high risks due to their specific circumstances which make them vulnerable countries. Our challenge: predict the vulnerability of the countries by 2030.
Given the crucial role of non-governmental organizations (NGOs) to address the effects of global warming on agriculture, our primary focus goes beyond identifying the most vulnerable countries, we aim to predict this vulnerability by the year 2030 and foresee the difficulties before they happen. In that way, we can empower NGOs with pivotal information that may serves as initiatives for them to take action and tailor solutions and strategies before the things get too hard for vulnerable countries.
A data-driven definition of Vulnerability
To achieve this ambitious goal, we delved into the world of data. This exploration involves carefully choosing our data from a reliable, open- data source: ‘Food and Agriculture Organization of the United Nations’. We focus on Geography and Economics data, Environment Temperature Changes Data and Agriculture (Crop Yield) Data.
- Geography and Economics data1: it underscores information related to percentage of the country area cultivated, Gross Domestic product (GDP), agriculture’s GDP contribution, total renewable water resources, and the national rainfall Index.
- Environment Temperature Change Data2: it focuses on temperature change factor for different countries.
- Agriculture (Crop Yields) Data3: it involves the production values for specific crop yields. All our datasets are meticulously organized, categorized by country and year.
Inspired by cutting-edge research45, we’ve strategically organized our variables into three main components. Each component serves as a building block for our mission to assess vulnerability in the face of global warming’s impact on agriculture. Let’s delve into these components:
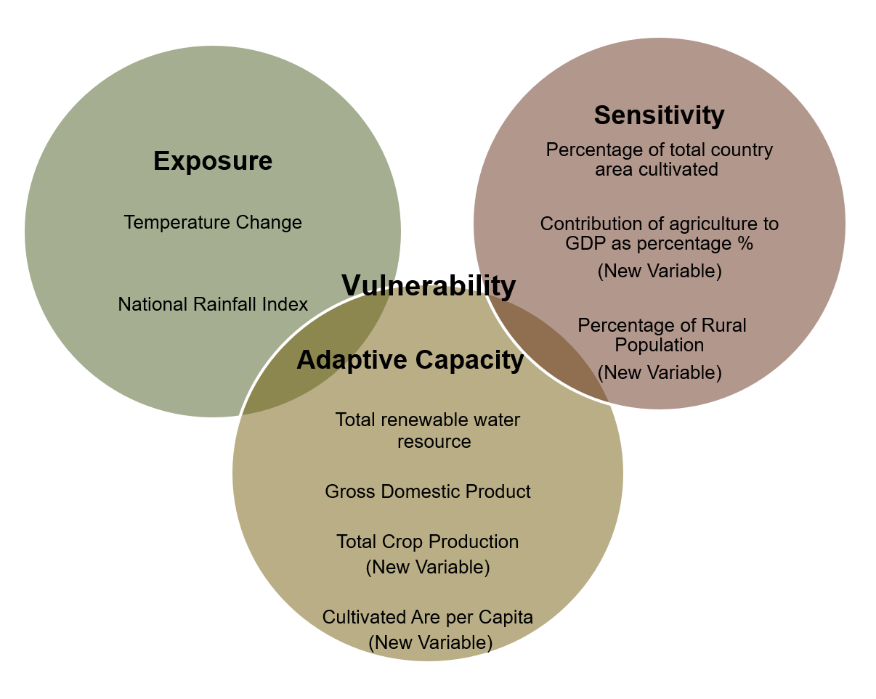
Exposure: How much the country is exposed to different climatic factors.
Sensitivity: How sensitive the agriculture in the area is to certain risks.
Adaptive Capacity: How well the area can adapt to and cope with the challenges posed by global warming.
We then attribute each variable to one of those three components as well as adding new variables to enhance our understanding.
Data Preparation
We started by preparing our datasets and transform them into refined insights in order to make them ready for future work.
- Rename the countries and matching them: We begin by renaming and identifying matching countries across all datasets. This is a foundational step when merging all the datasets together.
- Handling Missing Data: We address missing data using advanced techniques. The power of KNN imputation or zero imputation comes into play, ensuring our datasets are robust and comprehensive.
- Creating New Variables: we succeeded to create new variables as outlined in the data organizing phase. This step play an important role in shaping our analysis.
- Data Integration: Merging datasets together seamlessly, we ensure a unified view that enhances the effectiveness of our analysis.
- Data Normalization: Recognizing the diverse nature of our variables, we implement normalization techniques for both positive and negative variables. Positive variable indicates a positive relation with vulnerability, while negative variable signify a negative relation. This step ensures fair treatment of climate and agriculture variables for accurate vulnerability assessment.
Assessing Global Vulnerability: How does it Work?
Since our goal is to identify the vulnerable countries in the face of global warming’s impact on the agriculture by 2030, we embark in another journey that requires a deep comprehensive of methodologies for calculating vulnerability, making predictions and providing insightful vulnerability classifications.
Our proposal: a Vulnerability index
The key concept of assessing vulnerability of the countries was to create a new variable called ‘Vulnerability index’(VI). This calculation of this variable is based on two main approach that are taken from two innovative research.
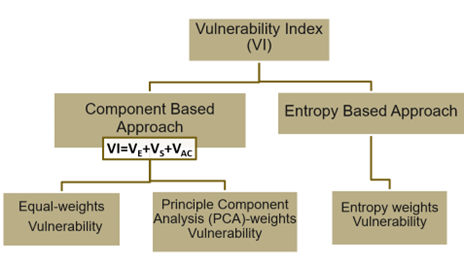
- Component -based Approach6:
As we organized our variables into three main components— Exposure(S), Sensitivity(S) and Adaptive Capacity (AC), we were seeking to calculate the vulnerability index by identifying the vulnerability of each component separately and than summing them up as illustrated in the figure. To achieve this, we assign weights to each variable attributed to one of these components. The weights represent the relative importance of each variable in the vulnerability index calculation.
We used two methods for assigning the weights: Equal weights method, where each variable is considered equally important and the Principle component Analysis (PCA) method, which involves mathematical techniques to capture the most critical information presenting in our data in a smaller set of components, then assigning weights based on the variables’ contribution to the first principal component. This dual-weighting strategy results in two different vulnerability indices: Equal -weights Vulnerability and Principle Component Analysis (PCA)- weights Vulnerability.
- Entropy- Based Approach7:
In contrast of the component-based approach, entropy-based approach simplifies the calculation of the Vulnerability Index by considering all the variables together. Measuring uncertainty and information content. The idea is to measure the uncertainty or information associated with each variable. This unique perspective on vulnerability, has a pivotal role in emphasizing variables that offer more certain and informative signals. We ended up after this approach, having Entropy-weights Vulnerability Index.
Making predictions
Having The three main Vulnrability indexes: Equal -weights Vulnerability and Principle Component Analysis (PCA)- weights Vulnerability and Entropy-weights Vulenrability, and recognizing the unique trajectories each country has followed, we decided to delve into the next phase: Prediction. In this step, we aim to predict our three vulnerability indices until the year 2030 by looking into the patterns and trends for each country. To achieve this, we used the ARIMA model, known as its suitability in short term-forecasting and capability in capturing temporal patterns8.
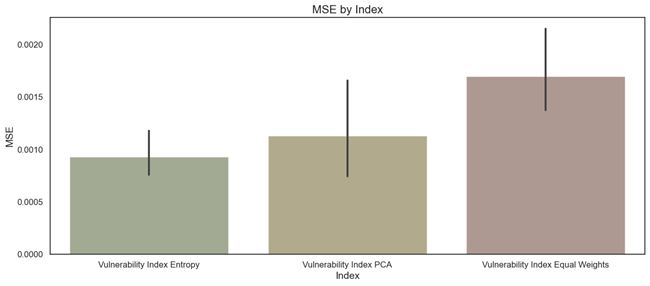
In order to evaluate the performance of the ARIMA model in making predictions for each of our vulnerability indices, we used the MEAN SQUARED ERROR (MSE) and MEAN ABSOLUTE ERROR (MAE) for evaluating our results. Thus, after exploring the results from the MSE measure from the figure below, we notice that the Entropy and PCA methods for calculating the vulnerability have a better performance than Equal Weights method. However, we can see that for all of those methods, we can consider that the error values are practically negligible.
Providing Vulnerability Classification
In this step, we looked forward to classify the countries based on the predictions’ values of each of their vulnerability indices. This involves categorizing countries based on their index values using the Percentile method. Countries below the 25th percentile of their vulnerability index is considered as Relatively non-vulnerable, those above the 75th percentile are considered as Relatively vulnerable , and the rest fall into Neutral Category. This classification provide us with a list of vulnerable countries for both 2020 and 2030 across all indices.
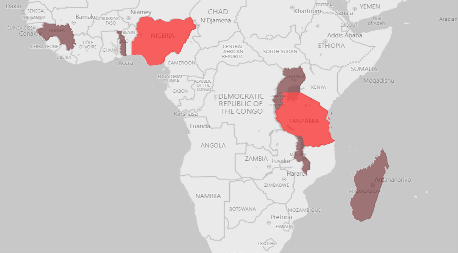
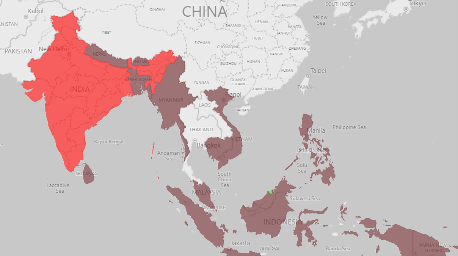
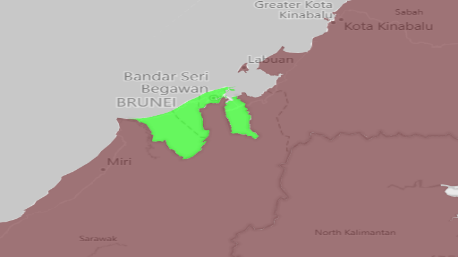
The figures above represent the vulnerable countries we got after extracting the common vulnerable countries from the classifications results of the three vulnerability indices. The countries in brown are the countries that remain vulnerable from 2020 to 2030, the red countries are the new countries that turn into vulnerable in 2030 and the green one is the country that improves its status from vulnerable to neutral by the year of 2030.
To see how the vulnerability index and the countries’ ranking have changed over years, we made an animated bar chart race to illustrate it. You can click here.
Turning Data into Action
As we navigate the impacts of global warming on agriculture, our journey has revealed insights into vulnerability across nations. We got a list of 24 vulnerable countries in 2020 and a list of 27 vulnerable countries in 2030 in which there are some countries that are going to be vulnerable in 2030 such as India, Nigeria, Tanzania and one country that will improve its status by the year of 2030: Brunei. With this information, we propose some strategies to the NGOs to take specific actions to support those countries indeed.
For those countries that remain vulnerable until 2030, NGOs can take some actions to improve their status by developing and implementing sustainable agriculture practices. This includes promoting efficient water management, soil conservation, and the adoption of climate-resilient crop varieties.
Countries that are going to be vulnerable by the year of 2030 need preemptive strategies. NGOs can collaborate to establish early-warning systems, support climate-smart agricultural practices, and facilitate knowledge exchange between nations that have successfully mitigated vulnerabilities. Moreover, NGOs can work on some factors in the Adaptive Capacity Components to avoid vulnerability like expanding cultivated areas, supporting population control measures, and boosting total production through research and innovation.
While our findings provide a valuable insights, acknowledging limitations is key. Expanding datasets, incorporating subjective insights, and focusing on specific crop vulnerabilities are our directions for future exploration. So let’s turn these improvements into actionable steps for a resilient and sustainable agricultural future.
- FAO AQUASTAT Dissemination System ↩︎
- FAOSTAT Temperature change on land ↩︎
- OURWORLDINDATA Crop Yields ↩︎
- Duong Thi Loi, Le Van Huong, Pham Anh Tuan, Nguyen Thi Hong Nhung, Tong Thi Quynh Huong, Bui Thi Hoa Man, An Assessment of Agricultural Vulnerability in the Context of Global Climate Change: A Case Study in Ha Tinh Province, Vietnam, 2022 ↩︎
- Lotten Wirehn, Åsa Danielsson, Tina-Simone S. Neset, Assessment of composite index methods for agricultural vulnerability to climate change, Journal of Environmental Management 156 (2015) 70-80, 2015. ↩︎
- Lotten Wirehn, Åsa Danielsson, Tina-Simone S. Neset, Assessment of composite index methods for agricultural vulnerability to climate change, Journal of Environmental Management 156 (2015) 70-80, 2015. ↩︎
- Leifang Li, Renyu Cao, Kecheng Wei, Wenzhuo Wang, Lei Chen, Adapting climate change challenge: A new vulnerability assessment framework from the global perspective, Journal of Cleaner Production, 2019. ↩︎
- https://machinelearningmastery.com/arima-for-time-series-forecasting-with-python ↩︎